Science & Intelligence Analysis
Intelligence analysis is a dynamic, interactive battle of wits played under complex, uncertain circumstances. Analysts must gather evidence from open and classified sources, evaluate its credibility, interpret its message, extract its implications, integrate it with other knowledge, synthesize an overall picture and communicate their conclusions along with the appropriate confidence. All the while, they know the world may be changing as friends and foes alter their strategies and tactics, partly through second-guessing one another’s actions as informed by intelligence analysis.
The vital role intelligence analysis plays in national security is reflected in the money, talent and lives invested in it. Much of that investment is in technologies, which provide unprecedented volumes, and sometimes kinds, of information. The importance of those technologies will only grow through advances in their capability and in the vulnerabilities they create (e.g., cybersecurity).
Ultimately, though, intelligence analysis is a human enterprise. Analysts must exercise judgment in deciding where to look for evidence, what they are seeing, what it means, what to pass on to different audiences, what cautions to include and what recipients will infer from different formulations of their message. Even when technology is essential, exploiting its potential requires inferences about human behavior. What patterns should the analyst be monitoring? Have those patterns changed in a meaningful way? Do those changes reflect what is happening or in what the analyst is able to observe? Are they being fed misleading information? Whom should the analyst consult? How does he/she get their attention? Whom should they tell? What should the analyst say and when?
Of course, intelligence analysts are not the only experts grappling with complex, uncertain, dynamic events. Consider, for example, pharmaceutical regulators wondering about telltale signs that a drug is not working as well as expected, public health officials puzzled by reports of young people dying inexplicably in Cambodia, bankers concerned that markets have lost the liquidity their financial models assume, severe storm forecasters asking if a derecho might be forming or electricity grid operators worried about storm forecasts. The work of these professionals parallels the analytical tasks faced in everyday life by parents helping their children (or their own aging parents), patients monitoring their health and investors managing their retirement portfolios.
The ubiquity and importance of decision-making under certainty have long made it a topic of scientific study. That research took off some 30 years ago with the confluence of what had been two semi-independent research streams. One stream, normative analysis, asks how people should make decisions.
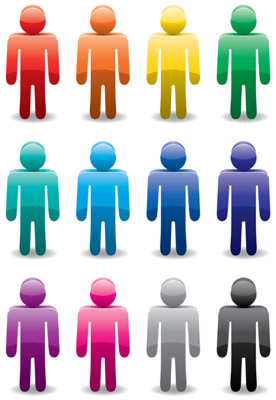
© iStockphoto.com/FreeSoulProduction
The second stream, descriptive research, asks how people actually do make decisions. The two streams came together when scientists developed ways to describe behavior in terms comparable to the analyses.
That allowed designing and testing prescriptive interventions for moving behavior closer to the normative ideal and making normative analyses more relevant to practical concerns. My February 2012 livebetter article, “Behaviorally Realistic Solutions to Environmental Problems,” describes that research as applied to everyday decisions focused on sustainability.
Recently, I had the honor of chairing a National Academy of Sciences committee, supported by the Office of the Director of National Intelligence (ODNI), tasked with assessing what social, behavioral and decision sciences had to say about intelligence analysis. Fortunately, veteran analyst Richards Heuer had been early to see this opportunity, resulting in his Psychology of Intelligence Analysis. Our committee’s work built on the foundation he helped to create. We produced a consensus report summarizing our analyses and a book of readings, making it easy to learn more about the topics we addressed.
Paraphrasing the report, we concluded:
1. The intelligence community’s “human capital” is its primary asset.
Ultimately, analysis depends on having the right people able to perform these difficult inferential tasks. As a result, effective intelligence organizations must recruit, reward and retain those people. That means having personnel policies that hire people with the needed stable individual attributes (e.g., cognitive ability, critical thinking, integrity), then focus their training and incentives on malleable attributes (i.e., job-specific knowledge, essential procedures).
The more fluid an organization’s external environment, the more important those stable attributes become. Individuals with those attributes are better able to adapt to new problems while taking advantage of their knowledge of how the organization works – where to find complementary expertise within it and how to meet its customers’ needs. We all have intuitions about how to evaluate those attributes in other people (e.g., deciding who is creative or trustworthy). Unfortunately, research finds that our intuitions are often bias prone. For example, “halo effects” can lead us to ignore flaws in generally strong candidates. In-person interviews give an illusory feeling of revealing who people really are. The science of personnel selection recommends looking instead at systematic measures of individuals’ behavior, such as prior work experiences and at scores on psychometrically validated tests.
Changing operating conditions can make it hard to find people with needed domain-specific skills. When organizations seek to reproduce themselves, they can turn to sources (e.g., military services, academic institutions, immigrant communities) that provided their current staff. However, with new topics (e.g., newly important technologies, cultures, measurement methods) they may not know where to look, what to look for or how to evaluate claims. One important source is graduates of research universities, who have been educated in a world attuned to the latest work that has survived rigorous peer review.
2. Analysts need strong tools.
Analytical research has identified a relatively small number of fundamental methods. Arguably, every educated citizen should know something about each. Certainly, every analyst should. Each method identifies the evidence relevant to understanding its class of problems and then provides a way to organize the evidence. As a result, using it reduces the chances that critical pieces of evidence are neglected or get lost along the path from collection to inference. Each method can be used for complex formal modeling. However, just knowing the logic of each can show how to structure important messy problems.
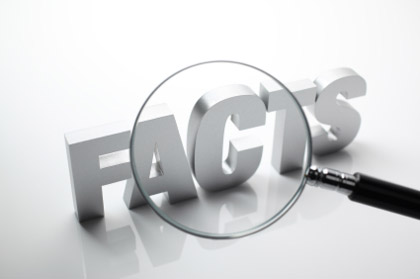
© iStockphoto.com/blackred
All that analysts need is the ability to tell when a method is relevant, how to apply it heuristically and when to seek professional help (for full applications). The committee’s book of readings offers intuitive introductions to these methods (unlike standard coursework emphasizing technical proficiency).
The methods include:
Signal detection theory characterizes the two properties of analysts’ judgment that determine the chances of their reporting an event (e.g., troop movement, cyber attack, equipment malfunctions). One is the analysts’ ability to pick out that “signal” from the “noise” surrounding the events. The second is the analysts’ confidence threshold for reporting an event, weighing the risks of true and false alarms. Knowing about signal detection theory helps organizations create procedures that distinguish perceptive analysts from trigger-happy ones.
Operations research predicts behavior of complex systems, such as supply chains, traffic flows, waiting lines and manufacturing processes. It identifies features critical to designing such systems – and to disrupting them. It can reveal unexpected simplicity, as with Little’s equation, showing the average length of a queue depends on just two things: how rapidly people (planes, etc.) arrive and how long it takes them to leave. It can also reveal limits of predictability. Knowing about operations research can both provide simple solutions and undue simplification, as when people interpret meaningless patterns in random processes (e.g., cancer clusters, sudden congestion in otherwise free-flowing traffic).
Decision theory organizes elements of a decision to allow identifying the best choice. Those elements are familiar: options, consequences and uncertainties (about which consequences will follow the choice of each option). Despite that familiarity, they can swirl around in the head unless one has a structured way to ask what people should do and where they can go astray. Knowing about decision theory focuses attention on the most critical issues while avoiding common oversights, such as neglecting opportunity costs of roads not taken and undue commitment to sunk costs.
Game theory structures analysis of interdependent decisions as friends and foes anticipate one another’s moves. It clarifies implications of parties properly and improperly, thus reading one another’s perceptions and objectives. It can look at a “single play” or multiple rounds of interaction. Knowing about game theory can reduce risks of exaggerating one’s ability to define the terms of engagement, overlooking opponents’ options for seizing the initiative and missing opportunities for compromise.
Each of these methods, like the others featured in our report, provides a disciplined way to ask natural questions. As a result, any analyst should be able to achieve the fluency needed to apply the basic concepts, converse with fellow analysts in these terms and describe problems to professionals. Relying on such proven methods provides protection against being sold unproven new ones, promising perhaps to do the impossible.
3. Analysts need opportunities for continuous learning.
Descriptive research has found that the intelligence community’s primary asset is, inevitably, an imperfect one. Analysts, like everyone else, are limited in what they know and in how well they assess how much they know. They can be over confident in their knowledge, moving too quickly and ignoring signs of trouble; they can also be under confident, gathering information unlikely to affect their choices. Analysts would be only human if they also tended to exaggerate how well they read the minds of people they are observing. And, conversely, if they exaggerate how well they have been understood by those listening to them.
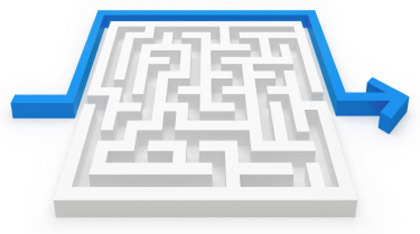
© iStockphoto.com/ssstep
People often cope with complexity and uncertainty by relying on shortcuts. Those include heuristic inferential rules (e.g., “they wouldn’t do something so out of character;” “we can’t plan for things that we can’t imagine”) and intuition (e.g., “this doesn’t feel right to me;” “I’ve seen this kind of situation get out of hand”). How well various shortcuts work under various circumstances is the subject of intensive study and controversy. Such contentiousness is essential to scientific progress: Researchers make claims, which are strong and clear enough to be tested and tempered by evidence as well as drawing critical scrutiny. However, it can be hard for analysts, who may feel forced to choose between ignoring research until scientists sort things out and putting undue faith into some overhyped pet theory.
In order to help analysts navigate the research, our committee summarized some of the relatively settled science. For emerging science, our report suggests the more analysts know, the better – as long as they recognize its status. Even emerging science can identify measures that might work (because they address known problems and build on known strengths) and ones that are likely to fail (because they require unintuitive ways of thinking). Whatever its status, however, no basic science can guarantee successful application. As a result, no substitute exists for evaluating how well any approach is working.
One standard method for evaluating judgments of uncertain events is assessing how well those judgments are calibrated. With well-calibrated ones, events given a 70 percent chance of happening actually happen 70 percent of the time. Many studies have found that individuals’ confidence is correlated with their knowledge. That is, they have a feeling for when they know more and less. However, they tend to exaggerate how well they can distinguish different states of knowledge. As a result, they sometimes have too much confidence and sometimes too little. The research also finds that calibration improves if people get prompt, unambiguous feedback with proper incentives – rewarding them for candor about how much they know rather than for bravado or hedging.
Such learning requires providing precise numeric probabilities, something that some analysts, like many other people, are reluctant to do. Research finds that people typically like getting precise predictions but hesitate to provide them. When that happens, as noted by Sherman Kent long ago (https://www.cia.gov/library/center-for-the-study-of-intelligence/csi-publications/books-and-monographs/sherman-kent-and-the-board-of-national-estimates-collected-essays/6words.html), their customers must press for the precise predictions their decisions require. Even when leaders act with great confidence, they need to know what gambles they are taking, as captured in the uncertainty assessments of well-calibrated analysts.
Conclusion
Our reports address many other issues, such as how to facilitate information sharing among analysts and promote healthy rivalries among working groups. In each case, current social, behavioral and decision science provides the point of departure for identifying problems worth addressing and solutions worth trying. Recognizing that all methods and analysts are imperfect, and that success in one context does not guarantee success in others, the report stresses the need for continuous evaluation. Effective intelligence organizations need to know the relevant science and test its implications for their reality – trying to learn faster than the competition.